Unlocking the Power of Data Labeling Tools for Your Business
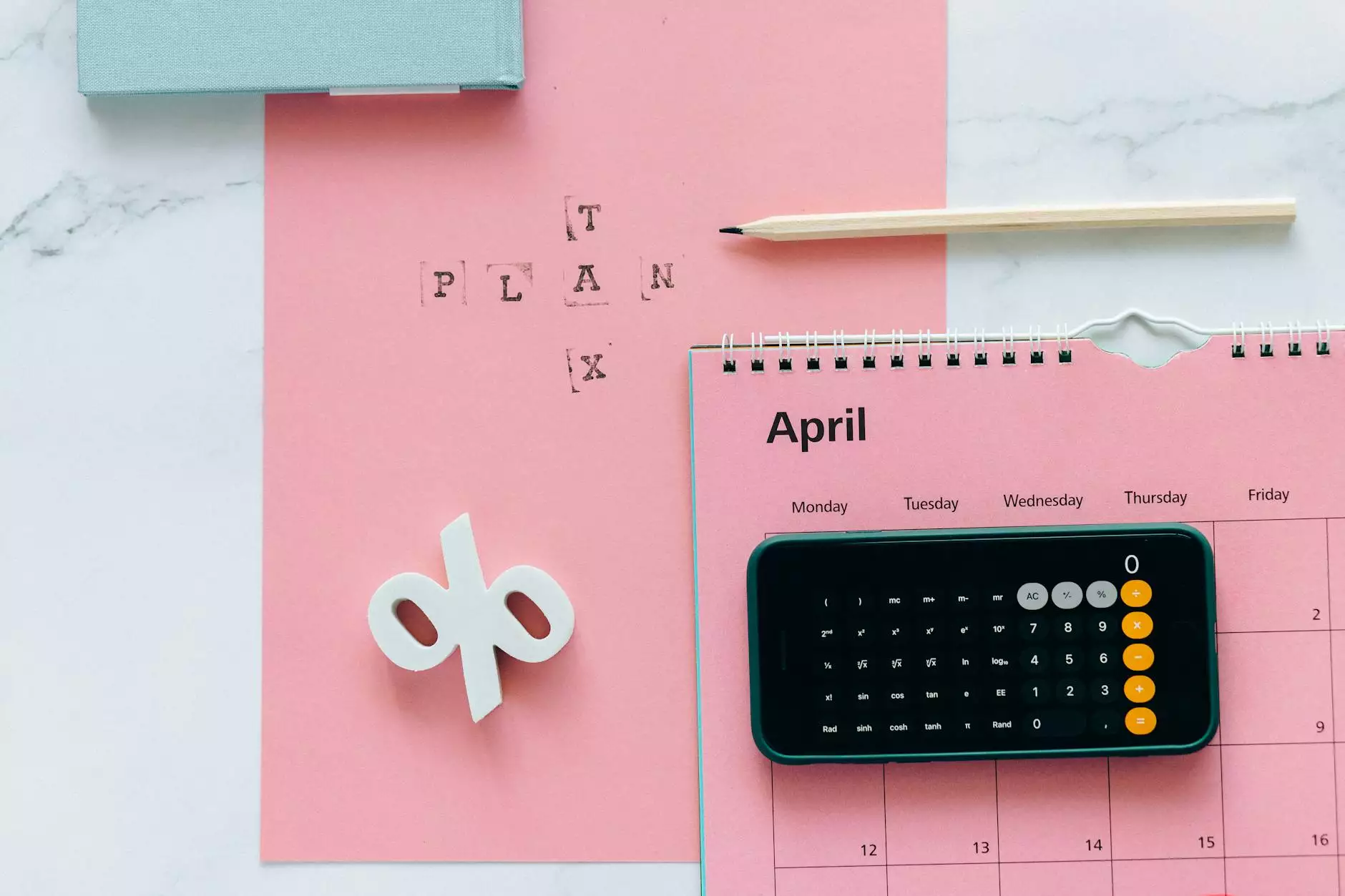
The digital landscape is rapidly evolving, and at the heart of this transformation is data. Businesses today are inundated with vast amounts of information that, when harnessed correctly, can lead to groundbreaking innovations and solutions. One of the crucial components of effectively utilizing data is through data labeling tools. In this article, we will delve deep into what data labeling tools are, their importance, how they streamline business processes, and how platforms like Keylabs.ai are revolutionizing this space.
What Are Data Labeling Tools?
At its core, a data labeling tool enables the annotation of raw data to create a structured and organized dataset that machines can easily interpret. This structured data is vital for training machine learning models, improving AI algorithms, and enhancing the overall quality of data analysis.
The Process of Data Labeling
The process of data labeling typically involves the following steps:
- Data Collection: Collecting raw data from various sources, including images, videos, text, and audio.
- Data Annotation: Applying tags or labels to the collected data based on predefined criteria.
- Quality Control: Reviewing the labeled data to ensure accuracy and consistency, which is vital for the success of machine learning models.
- Integration: Integrating the labeled data back into the machine learning pipeline for training and testing.
Why Data Labeling Tools Are Essential for Businesses
Businesses can no longer afford to overlook the significance of data labeling. Here are several reasons why investing in a data labeling tool is crucial for growth and innovation:
1. Improved Accuracy in Machine Learning Models
When it comes to machine learning, the accuracy of the outcome heavily relies on the quality of the data fed into the model. By utilizing advanced data annotation tools, businesses can ensure that their datasets are meticulously labeled. This attention to detail results in more precise predictions and better-performing algorithms.
2. Enhanced Operational Efficiency
Automation is a game-changer in any industry. A robust data labeling platform can streamline the entire data annotation process, saving time and resources. Automated labeling reduces the need for manual intervention, allowing your team to focus on more strategic initiatives rather than repetitive tasks.
3. Better Customization and Flexibility
Every business has unique data needs. Data labeling tools can be tailored to fit specific requirements, ensuring that the annotated data serves its intended purpose effectively. This customization leads to enhanced flexibility in adapting to changing market demands.
4. Scalability
As businesses grow, so does the volume of data they handle. A scalable data annotation platform allows organizations to manage increasing data volumes without compromising quality. This scalability is vital for enterprises looking to expand their operations seamlessly.
Types of Data Labeling Tools
Data labeling tools come in different forms, each tailored to specific types of data. Here are the most common types:
- Image Labeling Tools: These tools are used for annotating images and can be utilized for tasks such as object detection, image classification, and segmentation.
- Text Annotation Tools: Used for labeling texts and documents, enabling tasks like sentiment analysis, entity recognition, and categorization.
- Audio Annotation Tools: These assist in transcribing and labeling audio data for speech recognition and audio classification.
- Video Annotation Tools: Ideal for labeling video data, helping in tasks such as action recognition and frame-by-frame analysis.
Key Features of an Effective Data Labeling Tool
When considering a data labeling tool for your business, it's essential to look for specific features that enhance its efficacy:
1. User-Friendly Interface
A tool with an intuitive interface will reduce the learning curve for your team, allowing them to get up to speed quickly, which is crucial for productivity.
2. Collaboration Tools
Collaboration is key in any organization. A great data labeling tool facilitates teamwork, allowing multiple users to work on projects simultaneously, share notes, and provide feedback.
3. Quality Assurance Mechanisms
In-built quality checks ensure that the labels applied meet the required standards and eliminate errors that can lead to poor model performance.
4. Flexibility and Versatility
The tool should be adaptable to various types of data and suitable for different industries, allowing you to pivot as necessary without needing to retrain your team on new software.
The Role of Keylabs.ai in Data Labeling
Keylabs.ai stands at the forefront of data annotation technology. This platform offers cutting-edge solutions tailored to meet the diverse needs of businesses across multiple sectors.
Advanced Features of Keylabs.ai
Keylabs.ai provides a suite of advanced features that set it apart from other data labeling tools:
- AI-Powered Automation: Utilizing artificial intelligence for faster and more accurate data labeling.
- Customizable Workflows: Allowing businesses to create workflows that align with their specific data annotation needs.
- Robust Security Measures: Ensuring your data is secure with end-to-end encryption and strict privacy protocols.
- Real-Time Collaboration: Teams can work together in real-time, exchanging ideas and improving the labeling process.
Best Practices for Using Data Labeling Tools
To maximize the effectiveness of any data labeling tool, consider the following best practices:
1. Define Clear Labeling Guidelines
Establish comprehensive guidelines for annotators to follow. Well-defined rules minimize inconsistencies and enhance the quality of the labeled data.
2. Regular Training and Updates
Continuously train your team on best practices and updates to the labeling tool. Keeping your team informed allows them to utilize the tool's potential fully.
3. Implement Quality Control Processes
Regularly review labeled data to catch any mistakes early. Periodic audits can uncover patterns of error that need addressing.
4. Encourage Feedback and Communication
Fostering an open line of communication allows workers to share insights, challenges, and suggestions, leading to continuous improvement of the data labeling process.
Conclusion: The Future of Data Labeling in Business
The landscape of data utilization is evolving rapidly, and as businesses strive to keep pace, data labeling tools are undeniably becoming indispensable assets. With platforms like Keylabs.ai leading the charge, organizations can now effectively annotate their data, ensuring the highest quality for their machine learning models. By embracing effective data labeling practices, businesses can turn raw data into powerful insights that drive decision-making and foster innovation.
As we move further into an era dominated by artificial intelligence and data-driven decisions, investing in the right data labeling tools is not just beneficial; it is essential for long-term success and sustainability.